Generative AI Governace
Generative AI (GenAI) uses advanced algorithms to create new content like text, images, audio, and video by analyzing patterns in large datasets.
While it offers significant economic potential, businesses must address risks such as intellectual property concerns, bias, and compliance with evolving AI regulations like the EU AI Act through strong governance frameworks.
What is Generative AI?
Generative artificial intelligence (also Generative AI or GenAI), describes algorithms (such as ChatGPT) that can be used to create new content such as text, images, audio, music, and videos.
Generative models work by analyzing large amounts of data to learn patterns and then use that information to predict what would come next in a sequence.
GenAI applications are distinct from traditional AI in that they leverage machine learning techniques to create new, novel, content by understanding and mimicking the patterns of the training data. Traditional AI is primarily concerned with analyzing and making decisions based on input data without creating new content.
Economic Potential of Generative AI
Growth in the number of Gen AI projects is expanding rapidly. McKinsey estimates that Generative AI solutions could add the equivalent of $2.6 trillion to $4.4 trillion annually to global economic output. The interest and excitement associated with GenAI has been fueling the growth of not only generative models but also that of traditional AI models as well.
Top Generative AI Use Cases
- Chat Based Interfaces Embedded in Products
- Customer Communications and Contact Centers
- IT Automation & Cybersecurity
- Coding Assistants
- Knowledge Assistants (e.g. sales copilot)
- Content Development (e.g marketing personalization)
New Risks Specific To Generative AI
While GenAI offers enterprises the opportunity to create value by applying AI to a new class of problems, this opportunity does not come without risk. Generative models carry all of the risks inherent with traditional AI models that are also part of the Enterprise AI portfolio.
For example, they can have issues associated with fairness, bias, or safety.
General Risks of Generative AI
- Data Privacy and Security Concerns
Generative AI systems require extensive data for training, which often includes sensitive or proprietary information. Without robust data security measures, there's a risk of exposing trade secrets and customer data. For instance, inadequate AI or data governance can lead to unauthorized access or data breaches, compromising both company and client information (Joyce et al., n.d.). Gartner predicts that improper use of GenAI will be responsible for at least 40% of AI-related data breaches across the world by 2027 (Gartner, n.d.). - Intellectual Property (IP) Infringements
Generative AI models can unintentionally replicate existing content, posing risks of IP violations. For instance, if an AI system produces content that closely resembles a copyrighted work, it may lead to legal disputes, particularly in industries like media and entertainment, where originality is crucial (Lawton, 2024). A notable case occurred in 2023 when Samsung Electronics banned employees from using ChatGPT after discovering that some had inadvertently fed sensitive IP data into the system. By default, ChatGPT retains user data to improve its models unless users opt out, highlighting concerns around data security and intellectual property protection (Ray, 2023). - Bias and Discrimination
AI models trained on biased data can perpetuate or even amplify existing prejudices, leading to discriminatory outcomes. For instance, a generative AI used in recruitment might favor certain demographics if the training data reflects historical biases. Such biases can result in reputational damage and potential legal repercussions for organizations (“The flip side of Generative AI”, n.d.). - Generation of Misinformation
Generative AI has the capability to produce content that appears credible but is factually incorrect. This "hallucination" can lead to the dissemination of misinformation, which can mislead stakeholders and damage public trust (Lawton, 2024). For example, Air Canada’s chatbot promised a customer a discounted fare he did not qualify for. Air Canada said the chatbot is responsible for its own actions, but ultimately, the company had to pay the passenger $812.02 (Yagoda, 2024). - Regulatory and Compliance Challenges
The evolving regulatory landscape for AI poses compliance challenges for enterprises. The EU AI Act’s unacceptable risk deadline started February 2nd, 2025, and organizations must navigate regulations concerning data usage, privacy, and AI ethics. Non-compliance can result in legal penalties and hinder AI initiatives. For instance, adhering to data protection regulations requires diligent oversight of AI data handling practices (Joyce et al., n.d.). - Security Vulnerabilities
Generative AI systems can be susceptible to adversarial attacks, where malicious actors manipulate inputs to deceive the model into producing harmful outputs. For example, an attacker could input crafted data to cause an AI system to generate malicious code or misinformation (Lawton, 2024). Such vulnerabilities can compromise system integrity and security as seen when someone tricked a ChatGPT powered chatbot to sell a 2024 Chevy Tahoe for $1. - Operational Dependence and Reliability Issues
Over-reliance on generative AI can lead to operational challenges, especially if the AI system fails or produces unexpected outputs (Joyce et al., n.d.). Recently, Virgin Money's AI-powered chatbot mistakenly flagged the word "virgin" as inappropriate when a customer inquired about merging their Individual Savings Accounts (ISAs), leading to a frustrating interaction. This incident highlights the risks of AI misinterpretation in customer service, potentially harming user experience and company reputation (Quinio, 2025). - Inadequate AI Governance and Unclear Accountability at Companies
AI governance involves ensuring that the technology is used responsibly and transparently. Inadequate governance frameworks can lead to issues such as the misuse of AI for unethical purposes, lack of accountability in decision-making, or insufficient oversight. This could expose companies to legal challenges or undermine public confidence in AI technology (Committee of 200, 2025). A notable example highlighting the challenges of AI governance in the private sector involves OpenAI's internal management of its generative AI technologies. In November 2023, OpenAI's board of directors dismissed CEO Sam Altman, citing concerns over his lack of transparency and potential conflicts of interest related to AI safety processes. This incident underscores the critical need for robust governance frameworks to ensure responsible and transparent AI development, as inadequate oversight can lead to internal conflicts and undermine public trust in AI technologies (Perrigo, 2024)
- Ethical and Societal Implications
The deployment of generative AI raises ethical questions, including concerns about job displacement due to automation, the potential misuse of AI-generated content and denial of insurance claims (Lawton, 2024). Giant insurance companies like UnitedHealthcare, Humana, and Cigna are facing class-action lawsuits over their use of AI algorithms, which have led to a rising number of claim denials for life-saving care. As a result, enterprises must carefully navigate these ethical challenges to uphold public trust and fulfill their social responsibility (Schreiber, 2025).
Enterprise Risks of Generative AI
But beyond the fundamental risks that are common to all AI uses, GenAI and Large Language Models (LLM) in particular, introduce additional risk elements.
A few of the most significant incremental risks include:
- Democratization of Model Building - GenAI’s ability to understand natural language through the use of Large Language Models (LLM) introduces the potential for many more individuals (non data scientists) to create models that in turn require some level of oversight.
- Novel Content Creation
- Intellectual Property Leakage
- Copyright Infringement
How to Balance GenAI Innovation and Risk
Balancing the innovation potential of GenAI with its inherent risks requires a structured approach that prioritizes governance, compliance, and responsible deployment. Organizations must implement robust AI governance frameworks that ensure transparency, accountability, and security while enabling innovation at scale.
- Establish Visibility and Inventory of AI Systems
To mitigate risk, enterprises need a single source of truth for all AI initiatives. A comprehensive inventory of AI use cases and models enables organizations to track performance, identify risks, and enforce compliance in real time. - Automate Risk Controls and Compliance
Organizations should enforce standardized governance policies across AI projects. Automated workflows ensure regulatory adherence, ethical AI development, and consistent oversight, reducing legal exposure and operational risks. - Monitor and Mitigate Bias, Misinformation, and Security Threats
Continuous AI monitoring allows organizations to detect and address bias, misinformation, and adversarial threats. Implementing automated testing and validation frameworks ensures AI-driven decisions remain fair, accurate, and aligned with ethical standards. - Implement Responsible AI Safeguards Without Stifling Innovation
The key to balancing risk and innovation is integrating AI governance seamlessly into AI development workflows. Scalable governance models enable teams to experiment and deploy AI rapidly while ensuring compliance with evolving industry regulations. - Ensure Enterprise-Wide AI Accountability and Transparency
A clear AI governance structure fosters accountability across teams, ensuring AI aligns with business objectives and regulatory expectations. Real-time reporting and risk assessments provide executives with the insights needed to make informed decisions about AI investments.
By embedding governance into every stage of the AI lifecycle, organizations can confidently scale Generative AI while mitigating financial, legal, and reputational risks.
The Need for AI Governance
Increasingly GenAI is becoming tied to business revenue generation and cost reduction initiatives. Just as with traditional AI model development, businesses will need to manage the risks associated with generative models by putting in place an effective AI governance framework.
The regulatory trend is clear. A significant number of AI specific guides and regulations have been published in the last 5 years. It is a near certainty that the AI efforts of enterprises, both traditional and generative, will increasingly be the focus of audits and potentially significant penalties for non-compliance.
For example, failure to comply with provisions of the EU AI Act as it relates to what the act defines as high-risk AI uses, can result in fines up to 20 million euros or 4% of turnover.
Good Governance - Not Just Compliance
AI governance is not only important to ensure compliance with relevant AI regulations. Ensuring that Generative AI applications produce valid and accurate results is also a key benefit of good governance.
Consider the experience of Air Canada which launched a virtual assistant to help improve the customer experience and lower customer service costs. Chat bots of this type have become one of the most widely taken on-ramps for enterprises starting GenAI initiatives.
In a widely reported incident that occurred in 2022, Air Canada’s virtual assistant told a customer that they could buy a regular priced ticket and apply for a bereavement discount within 90 days of purchase.
When the customer submitted their refund claim, the airline turned them down, saying that bereavement fares couldn’t be claimed after ticket purchase. The customer took the airline to a court, claiming the airline was negligent and misrepresented information via its virtual assistant.
The court found in favor of the plaintiff and ordered Air Canada to reimburse the customer for the full price of the tickets. While the dollars involved in this example were not significant, it is easy to imagine scenarios where the financial risk could have been much greater.
Implementing SafeGuards for Generative AI
Large Language Models and Generative AI have massive transformational potential, so Corporate Boards and C-Level executives are putting enormous pressure on business, analytics, and IT leaders to quickly leverage this technology to improve revenue generation, increase customer satisfaction, and drive efficiencies. However, AI —and especially Generative AI — presents multiple socio-technical challenges that must be addressed to avoid financial, regulatory, and brand risk exposure.
Enterprise AI leaders are challenged to urgently navigate three major trends:
- Corporate boards are hyper-focused on using Generative AI, especially for customer service
- Executives need to protect the company from the brand, financial, and regulatory risks associated with Generative AI
- Engineering, finance, marketing, and sales are swiping credit cards to use third-party Generative AI tools like ChatGPT, Llama2, Claude, Bard, and Titan
Furthermore, there’s unprecedented support from academic institutions, governments, technology vendors, and citizens for regulations that provide guardrails for the safe and humane use of AI. From the EU Artificial Intelligence Act to the US NIST AI-Risk Management Framework and industry-specific guidance such as the Canadian OSFI E-23 Extensions for AI, the regulations will only continue to expand and evolve.
The bottom line is clear — enterprises can’t afford to wait to tackle AI Governance — the transformational potential is real, but the risks are already exposed within organizations.
That’s why ModelOp, the leader in AI Governance software for enterprises, released the latest version of ModelOp Center: to safeguard the use of Large Language Models (LLMs) for the entire enterprise.
ModelOp Enables AI, Risk, Compliance, Data, and Security Leaders To Accelerate Innovation with Generative AI
ModelOp Center version 3.2 is the first commercially available software that enables enterprises to safeguard Large Language Models (LLMs) and Generative AI without stifling innovation. 3.2 builds on ModelOp’s existing governance support for all models — including regressions, Excel, and vendor models — by adding new capabilities to manage LLM ensembles, track value, chart and visualize risks, provide universal monitoring, and enforce governance controls through model life cycle automation.
Supporting Generative AI Use Cases in a Fiscally Responsible Way That De-risks the Enterprise
The era of enterprise AI is here and companies are diving into Generative AI. According to Bain & Company over 40% of businesses are adopting or evaluating the top six applications for Generative AI:
- Chat-based interfaces embedded in products
- Coding assistants
- Customer communications / contact centers
- Knowledge assistants (e.g. sales copilot)
- IT automation / cybersecurity
- Content development (e.g. marketing personalization)
Generative AI is a catalyst for AI Governance. The innovative capabilities released in ModelOp Center version 3.2 were strategically built based on feedback from ModelOp customers who are dealing with the urgent issues surrounding both LLMs and more traditional models that have long powered decision-making in global enterprises.
Track Value and Visualize Risks
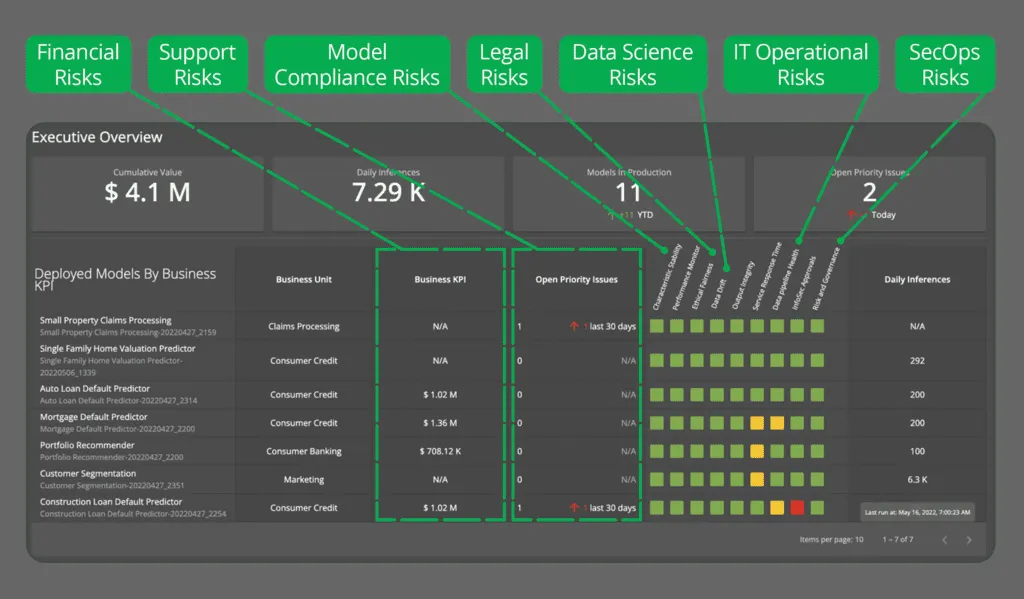
While LLMs offer enterprises transformational opportunities, they also need to be carefully tracked from a financial, data science, legal, security, and IT perspective, as there are many moving pieces that can introduce risk to the organization. ModelOp Center provides comprehensive, real-time insight into all facets of LLMs to present a true “Portfolio View” of an enterprise’s AI investments.
Track AI Usage and Monitor Production Risks
Version 3.2 adds support for comprehensive LLM Usage Tracking and Monitoring, including:
- Inventory: support for classifying and tracking LLM use cases and models in the ModelOp Center Governance inventory, including the ability to govern LLM ensembles
- Generative AI Asset Management: support for prompt templates, RAILS, etc. for Generative AI asset tracking
- Monitoring: support for Automated Production Monitoring of LLM related metrics, including sentiment analysis, PII/PHI analysis, and cross-LLM performance analysis
Enforce Controls Through Workflow Automation
ModelOp Center version 3.2 extends the existing governance workflow and controls enforcement to support LLMs and Generative AI, including:
- Risk Tiering: automated rules for proposing risk tiering based on key model inputs
- Governance Workflows: out-of-the-box regulatory workflows to support LLM specific testing and other gates
- Controls Check: support for Excel-based attestation of controls, to support LLM/AI-specific policies
- Generative AI Testing: automated testing & documentation generation for LLM specific metrics
Take Advantage of Pre-built Templates and Out-of-the-box Tests
ModelOp Center version 3.2 extends its vast set of out-of-the-box capabilities by adding the following:
- Out-of-the-box Governance Workflows: specific LLM checks, such as ensuring that all LLM packages contain prompt templates and guardrails as part of the model package
- Out-of-the-box LLM Tests and Monitors
- Out-of-the-box LLM Review/Validation Reports
Enterprises Cannot Wait Any Longer to Establish AI Governance
AI governance is way more than model monitoring, ethics, and fairness. ModelOp’s AI governance platform delivers the critical capabilities needed to inventory, control, and report on all models – including LLMs – across your enterprise.
Watch this short demo video on how you can use ModelOp to govern LLMs, including how to:
- Accelerate innovation and measure model ROI
- Enforce AI guidelines that de-risk the enterprise
- Achieve significant faster time-to-value and lower total cost of ownership than building in-house solutions
References
- KPMG. (n.d.). The flip side of generative AI. https://kpmg.com/us/en/articles/2023/generative-artificial-intelligence-challenges.html
- Committee of 200. (2025, February 4). AI governance: The CEO’s ethical imperative in 2025. Forbes. https://www.forbes.com/sites/committeeof200/2025/02/04/ai-governance-the-ceos-ethical-imperative-in-2025/
- Gartner. (n.d.). Gartner predicts 40% of AI data breaches will arise from cross-border GenAI misuse by 2027. https://www.gartner.com/en/newsroom/press-releases/2025-02-17-gartner-predicts-forty-percent-of-ai-data-breaches-will-arise-from-cross-border-genai-misuse-by-2027
- Joyce, S., Kashifuddin, M., Kosar, J., Persons, T., Agarwal, V., & Greenstein, B. (n.d.). Managing the risks of generative AI. PwC. https://www.pwc.com/us/en/tech-effect/ai-analytics/managing-generative-ai-risks.html
- Lawton, G. (2024, July 23). Generative AI ethics: 8 biggest concerns and risks. TechTarget. https://www.techtarget.com/searchenterpriseai/tip/Generative-AI-ethics-8-biggest-concerns
- Lopez, J. (n.d.). GM dealer chatbot agrees to sell 2024 Chevy Tahoe for $1. GM Authority. https://gmauthority.com/blog/2023/12/gm-dealer-chat-bot-agrees-to-sell-2024-chevy-tahoe-for-1/
- McCarty Carino, M. (2024, April 8). Pre-employment tests are getting the AI treatment. Marketplace. https://www.marketplace.org/2024/04/08/tech-is-supercharging-pre-employment-personality-tests/
- Perrigo, B. (2024, September 5). Helen Toner: The 100 most influential people in AI 2024. Time. https://time.com/7012863/helen-toner/?
- Quinio, A. (2025, January 29). Virgin Money chatbot scolds customer who typed “virgin.” Financial Times. https://www.ft.com/content/670f5896-1fe5-4a31-b41f-ad4f5b91202f
- Ray, S. (2024, February 20). Samsung bans ChatGPT among employees after sensitive code leak. Forbes. https://www.forbes.com/sites/siladityaray/2023/05/02/samsung-bans-chatgpt-and-other-chatbots-for-employees-after-sensitive-code-leak/
- Schreiber, M. (2025, January 25). New AI tool counters health insurance denials decided by automated algorithms. The Guardian. https://www.theguardian.com/us-news/2025/jan/25/health-insurers-ai
- Yagoda, M. (2024, February 23). Airline held liable for its chatbot giving passenger bad advice: What this means for travelers. BBC News. https://www.bbc.com/travel/article/20240222-air-canada-chatbot-misinformation-what-travellers-should-know
Govern and Scale All Your Enterprise AI Initiatives with ModelOp Center
ModelOp is the leading AI Governance software for enterprises and helps safeguard all AI initiatives — including both traditional and generative AI, whether built in-house or by third-party vendors — without stifling innovation.
Through automation and integrations, ModelOp empowers enterprises to quickly address the critical governance and scale challenges necessary to protect and fully unlock the transformational value of enterprise AI — resulting in effective and responsible AI systems.
To See How ModelOp Center Can Help You Scale Your Approach to AI Governance