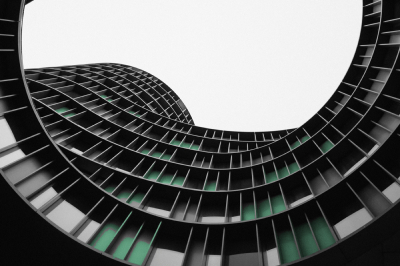

Dave Trier, Global Banking & Finance Review – March 4, 2021
In recent years, the banking industry has been at the forefront of AI and ML adoption. A recent survey by Deloitte Insights shows 70% of all financial services firms use machine learning to manage cash flow, determine credit scores, and protect against cybercrime. According to an Economist Intelligence Unit adoption study, 54% of banks and financial institutions with more than 5,000 employees have adopted AI.
But AI and ML adoption has not been easy. Difficulty in deployment has been exacerbated by the growing number of new AI platforms, languages, frameworks, and hybrid compute infrastructure. Add to this the fact that models are being developed by staff in multiple business units and AI teams, making it difficult to ensure that the proper risk and regulatory controls and processes are enforced.
As these AI initiatives and models multiply, risk managers and compliance officers are challenged to ensure proper governance measures are in place, and more importantly, adhered to. Without an auditable process, model risk management steps are often overlooked by those responsible for developing, monitoring, and governing models. If left unattended, steps are skipped leaving companies exposed to unacceptable business risk such as fines, unreliable model outcomes and, depending on model use, fraud.
Yet enforcing governance and risk requirements is a constant challenge, and one that is a delicate balancing act between enforcing risks while continuing to encourage innovation. As AI and ML adoption grows and regulatory guidance changes, monitoring and governance becomes more complex.
Here are five best practices that banks and financial institutions should consider following to ensure that AI and ML models are governed and monitored effectively.
1. Define an end-to-end model operations process
An end-to-end model operations process, referred to as a model life cycle (MLC) is a detailed workflow with well-defined steps for operationalizing and maintaining the model throughout its production life, from deployment to retirement. This includes steps for running and monitoring the model to ensure it continuously produces reliable results, as well as the steps a company has identified for controlling risk and adhering to regulatory and compliance requirements.
A model life cycle typically includes workflows for model registration, business approvals, risk controls enforcement, and model retraining, re-testing, re-validation, and eventually retirement. It ensures that the appropriate controls are put in place early in the operationalization process and should include thresholds that are identified and agreed upon with the 2nd line teams.
These workflows should integrate with existing applications, like data platforms, model development applications, IT service management systems, MRM systems, etc. instead of duplicating or replicating efforts. This will ensure that the latest information is being used in the model operations process and eliminate redundancy that often leads to inconsistencies.
The model life cycle establishes the technical and organizational scaffolding that unites data scientists, data engineers, developers, IT operations, model operations, risk managers and business unit leaders through clearly defined processes and ensures that all models are following the proper risk and governance procedures.
2. Register all models in a central production model inventory
The first step in operationalizing a model is registering the model(s) and associated artifacts in a centralized production model inventory. All the elements that compose the model—such as source code, tests, input and output schemas, training data, metadata, as well as outputs of training—should be included, along with all the elements required to execute it, including libraries.
With a growing number of different business processes and applications that use models and platforms that run models, it is increasingly challenging for IT and business executives to confidently have a pulse on what models are actually being used for business decisioning and where they are being used.
A centralized production model inventory provides visibility into all models running in production, regardless of where they’re executing, the business process or application they're serving, or the AI/ML language or framework used for development. This provides the flexibility to leverage existing investments, while still providing the proper level of controls for these critical business decisioning assets.
3. Automate model monitoring and orchestrate remediation
Monitoring begins when a model is first implemented in production systems for actual business use and continues until the model is retired. While most of the buzz in the AI world focuses on data drift and model accuracy, model risk teams need more comprehensive monitoring focused on population stability, characteristic stability, rank order break, score concentration, selection curves, model expiration dates, ethical fairness, and many others. AI models require more frequent monitoring based on shifts in data, ongoing enforcement of business and risk thresholds and other factors.
Detecting a problem is just the first step. To achieve optimal performance and reliability, remediation must be part of the monitoring process. Monitoring workflows need to include gathering problem information, obtaining performance metrics, generating reports for aiding in diagnosis, initiating and routing incident and change requests, taking corrective actions, gating activities that need approvals and tracking the entire process until model health and performance is reinstated.
For monitoring to be most effective, it should include alerts and notification of potential upcoming issues, and most importantly, it should be automated. With the speed at which AI and ML models are being developed and embedded into core business processes, monitoring models has grown beyond human scale in most companies.
4. Establish regulatory and compliance controls for all models
Models are a form of intellectual capital that should be governed as a corporate asset. They should be inventoried and assessed using tools and techniques that make auditing and reporting as efficient as possible.
The “black box” characteristics of AI and ML algorithms limit insight into the predictive factors, which is incompatible with model governance requirements that demand interpretability and explainability.
Many companies are attempting to extend their model risk processes for 1st and 2nd line teams, which is a great start, but consistent processes and automation are also required. While the entire governance process may not be able to be automated, it can be automatically orchestrated to ensure that all regulatory and business controls are enforced for all models and all steps are tracked, reproduceable and auditable.
Compliance and auditability require a systematic reproduction of training, evaluation and scoring of each model version and ultimately the transparency and auditability typically required for regulatory and business compliance.
5. Orchestrate, don’t duplicate or replicate
Automating and orchestrating all aspects of model operations ensures model reliability and governance at scale. Each model in the enterprise can take a wide variety of paths to production, have different patterns for monitoring and various requirements for continuous improvement or retirement.
A well-designed model operations process leverages, not duplicates, the capabilities of the business and IT systems involved in developing models and maintaining model health and reliability. This includes integrating with model development platforms, change management systems, source code management systems, data management systems, infrastructure management systems and model risk management systems. This integration provides the connection points for orchestrating actions, streamlining the model operations processes and allowing for end-to-end management of the complete model lineage that is traceable and auditable.
Making it all work
Technology is an important component for establishing good model operations and providing the responsiveness, auditability, and scalability that is needed, but it is not a magic bullet. Successful model governance requires significant collaboration between first line managers, risk managers, program managers, data scientists in the business lines, and the finance function for all the regulatory and capital reserve models as well as risk in technology.
AI governance and risk management will continue to evolve as AI models and technology change. Regardless, the model operations process must be properly defined, monitored and governed to produce the right business outcomes, which requires a combination of technology, well defined processes and a cross-team collaboration.