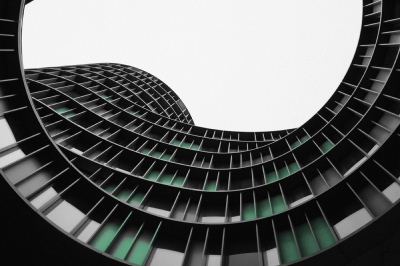
In my last post, we talked about the various AI regulations and guidance that have recently been published within the US and globally. Since then, the EU AI Act was approved by the European Parliament and will likely be ratified into law by the end of May. Couple these regulations with the real-world horror stories of “where AI has gone wrong,” and global enterprises have no choice but to implement an AI Governance program now.
But how can organizations get started?
Let’s be honest: many equate governance with “process overhead” or “big brother” watch dogs, often stalling innovation and reducing productivity. So how does an enterprise get started with the right level of governance to protect the organization, without stymying innovation?
In this post, we’ll cover a concept called “Minimum Viable Governance,” which aims to provide just enough governance for enterprises beginning their AI journey. For those with a Product Engineering background, this concept is similar to Minimum Viable Product (MVP), which is a version of a new product that allows the team to gather the maximum amount of proven customer knowledge with the least amount of effort.
Similarly, a company commencing their AI journey is determining where AI drives the most value to their customers, employees, organization, etc. Why would you not have an AI Governance capability (which is required for successful AI) that follows the same MVP approach?
Key elements of Minimum Viable Governance (MVG)
Regardless of whether you agree with calling it MVG, there are a few bare minimum capabilities required for AI Governance within an enterprise. Let’s examine these:
1. Governance Inventory
"You can't govern what you don't see."
If you don’t know about it, how can you govern it?
Whether it’s the EU AI Act, Texas House Bill 2060, or OCC SR11-7, the foundation of any governance capability is an Inventory, which provides visibility into everywhere that AI is being used.
While organizations and their leaders may realize they’re responsible for governing proprietary AI and models that are developed in-house, many are not aware that they’re also responsible for avoiding and mitigating the risks associated with third-party vendor (e.g. AWS Bedrock or ChatGPT) and Embedded AI (e.g. Salesforce Einstein or Epic Electronic Health Records (EHR)).
For instance, in the case of Healthcare, the World Health Organization (WHO) recently released AI ethics and governance guidance for large multi-modal models. According to WHO, healthcare providers that use software with AI are accountable if they cause harm by using the tool in inappropriate settings — such as for specific types of diagnosis or clinical care — because of training data bias or contextual bias that can result in avoidable errors and harm from known risks.
The point is you can’t solely rely on vendors to mitigate or be accountable for AI-related risks in their software or models. Organizations that leverage the technology are at risk and accountable too.
Specific Capabilities
Specifically, an MVG inventory should include:
- Overview information: AI name, description, scope, usage, limitations
- Accountability: business owner, model owner, IT owner, legal/compliance owner
- Risk Information: materiality, complexity, exposure
- For the EU AI Act, should include the categorization of Unacceptable Risk, High-Risk, Limited or Low-Risk
- Data information: data classification (including PII, PHI, etc.), data sets used for training/testing/validation (as appropriate), source systems
- Technical assets: source code, trained artifacts, endpoints (if vendor), system information (if SaaS/embedded AI)
- Documentation: the design documents, review documents
- Approvals: for traceability and accountability, the specific approvals by Business, IT, Legal/Compliance, etc.
- Risks: identification and tracking of the risks associated with the model and the appropriate mitigation
Pitfalls
- Manual Approach / DIY. Many think they can start with an excel spreadsheet or some other home-grown tracking mechanism. They run into the following inevitable challenges:
- The variety of different AI systems–open source, proprietary, cloud, vendor–models make it difficult to manage within an inventory, especially the technical assets and associated risks.
- The volume of disparate teams using AI make it impossible to keep pace with the demand for AI systems to be inventoried.
- Existing Tooling. Others think that their data science workbench (including cloud workbenches) already has an inventory, so “I’m covered!” The reality is that:
- These workbenches are designed for model development and thus are focused on building models, not governing them.
- Governance, by design, should be separate and independent from model development.
- Typically, these workbenches do not support all types of models — especially vendor and embedded AI, as the vendor’s goal is to control all development within the workbench.
The MVG Way
To get started with MVG, we recommend the following:
- Implement a systematic inventory immediately, as the pace of innovation and volume of demand will overwhelm any homegrown or manual system.
- Choose a vendor that has best practices already in place, instead of trying to learn everything from scratch.
- Start with a few simple integrations to streamline the process as much as possible for model owners, while ensuring that the MVG does not become a multi-year program.
2. Light Controls
"You can't let the students grade their own papers."
While we want to trust employees to do the right thing, there are many cases where they just don’t know the right policy or process. Thus, the second key element of MVG is a light controls framework that ensures (dare I say “prompts”) the various users to provide what is needed to safeguard the organization.
Specific Capabilities
Specifically, an MVG inventory should include:
- Ability to enforce the fundamental steps that must be followed for all AI systems.
- Per the EU AI Act (and many others), the required steps should follow a risk-based approach, meaning that more scrutiny should be applied for high-risk systems vs. low-risk systems. The MVG needs to allow for different control enforcement based on the risk tiering.
- While the controls will vary, they typically might involve ensuring that:
- The inventory record is fully populated
- All technical assets have the appropriate traceability
- There is the appropriate data set traceability
- There is no human tampering
- The appropriate documentation is completed
- The requisite independent reviews and approval are received
- There is a risk mitigation plan for any identified risks
- There is a fallback plan, should AI “go wrong”
- Auditability that all controls were followed
- Support for all different types of models: open source, cloud based, proprietary, vendor, embedded AI
Pitfalls
DIY. Again, organizations think this can be built in-house. After millions and years in investment, they realize that they can't keep pace with changes in (a) regulations and (b) technology.
The MVG Way
To get started with MVG, we recommend the following:
- Select a vendor that provides an automation approach to enforcing controls, as the controls need to be enforced across every team and every business unit in an organization (i.e. it needs to scale).
- Leverage baked in best practices, but start with the minimum required checks before introducing more comprehensive controls.
3. Reporting
At every Board of Directors meeting, they are asking two questions: (1) "What are we doing with AI," and (2) "How are we safeguarding the company?"
Given the transformational value of AI, every CEO, board of directors, and even shareholders are asking those two questions: (1) “What are we doing with AI,” and (2) “How are we safeguarding the company?”
Thus, the final key element in MVG is the ability to answer those two questions quickly and easily with intuitive reporting.
Specific Capabilities
Specifically, an MVG reporting capability should include:
- Ability to identify all AI systems by risk tiering, location, and ownership
- Capabilities to report on high-priority risks across the AI portfolio
- Automated metrics generation or ability to import existing metrics for each individual AI system
- Standard mechanisms to report on a governance or risk score to allow for comparison across the different types of AI
- Export capability, since every enterprise loves a BI dashboard
Pitfalls
Manual Reporting. The current status quo is for each team to create their own report, which gets sent to their manager, who tries to consolidate and roll it up. This snowballs into a state where there is no uniformity across teams or business units, leading to inaccurate reporting on usage and, more importantly, risks for the AI systems.
The MVG Way
To get started with MVG, recommend the following:
Assuming that MVG tenet (1) and (2) were completed, Reporting should come easy. However, it is paramount that executives align on:
- The key risks factors that they need surfaced to manage the business.
- Enforcing that the lightweight controls and associated processes be followed if any team wants to use AI.
Conclusion
AI Governance is no longer a nicety — it is a necessity, and it certainly doesn't have to be a hindrance. Getting started with an MVG approach to AI Governance will help strike the balance between delivering on the value of AI quickly, while safeguarding the organization from the inherent risks of AI.