Guest
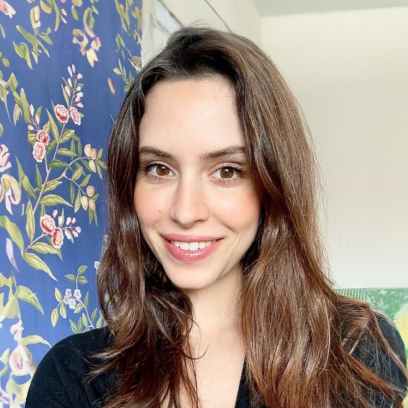
Watch Full Episode
Jay Combs: Hello, and welcome to the second episode of the Good Decisions podcast, ModelOps' AI governance insights podcast. I'm your host, Jay Combs, VP of Marketing at ModelOp. Today, we have Cristina Morandi, Director of Customer Success at ModelOp, who works closely with all our customers and is well-versed in the trends and happenings in AI governance. Welcome, Cristina.
Cristina Morandi: Hey, Jay. Thanks for inviting me. Glad to be here.
Jay Combs: It's great to have you. In our first episode of Good Decisions, we talked about risk tiering and its importance in AI governance.
Understanding Model Life Cycles
Jay Combs: We touched on how risk tiering impacts model life cycles and workflows. Today, we’ll dive into model life cycles. Let's start with a straightforward question: What is a model life cycle, and why is it important?
Cristina Morandi: That’s a great question, Jay. It’s also a loaded one because we need to be on the same page about what a model is. At ModelOp, we cast a wide net when referring to models, encompassing AI initiatives, statistical models, mathematical models, and rules-based decision-making models. Examples range from simple spreadsheets with calculations to complex machine learning models, in-house or vendor models, generative AI, LLMs, neural nets, and more. Regardless of complexity, it’s crucial to have a model life cycle in place.
The model life cycle is essentially the workflow a model undergoes from ideation to retirement. It includes development, testing, monitoring, controls, and reporting. Anything you do with the model should be well-understood.
Importance of Model Life Cycles for Businesses
Jay Combs: Why does a business need a model life cycle? Why is it important for a larger enterprise?
Cristina Morandi: Modeling and AI initiatives are expensive, whether developing or purchasing models. To maximize value from these investments, a plan is essential. There’s also significant risk associated with models, including how they’re used and their exposure. For higher-risk models, understanding the necessary requirements is crucial. Businesses need to grasp the full model life cycle to ensure models are compliant and operational without issues, with proper controls and monitoring in place.
Consistency and Compliance in Model Life Cycles
Jay Combs: You mentioned requirements, controls, and regulations. Is it fair to say that creating consistency for compliance across a large enterprise is a major part of this?
Cristina Morandi: Absolutely. Consistency in governing different model types is vital, especially for high-risk, customer-facing generative AI models. Consistent governance ensures that policies are enforced uniformly across the enterprise. Different model types or initiatives might have varying checks and balances, so a risk-based approach is crucial for the workflows.
Complexities of Model Life Cycles
Jay Combs: This sounds complex. Can you give insights into where these life cycles get complex in real-world organizations and their impacts?
Cristina Morandi: Complexity arises from two areas: the model life cycle itself and the volume of models. Model life cycles can have numerous control points and steps, requiring different groups’ involvement. For instance, a year ago, our customers had life cycles with 12-20 steps; now, we see over 300 control points. Managing these manually becomes impractical. Additionally, the volume of models has increased dramatically. Customers managing a dozen models a year ago are now dealing with hundreds, making manual processes unfeasible without automation.
Challenges of Manual Processes and Integration
Jay Combs: This isn’t just a data science team issue, right? It involves various teams like compliance, risk, legal, infosec, and data privacy. How do organizations scale from managing a few models to hundreds?
Cristina Morandi: Having the right tools is crucial. Automation is essential for monitoring, controls, testing, validation, ongoing reviews, and other risk management components. Integrating with other systems for processes and notifications is vital. Manual processes are unsustainable with increased AI investments and the need for disparate systems. Tools that are vendor-locked or specific to certain model types won’t work for broader AI investments.
Getting Started with Model Life Cycle Documentation
Jay Combs: When should enterprises start documenting the model life cycle and controls?
Cristina Morandi: Immediately. As soon as you have an idea, start defining the life cycle. Without a proper workflow, you risk wasting investments in models that can’t be used due to non-compliance. Early documentation ensures that models can move through the life cycle smoothly and deliver value.
Lessons and Insights for Model Life Cycle Definition
Jay Combs: What lessons or insights have you gathered from working with Fortune 500 companies on model life cycles?
Cristina Morandi: Key takeaways include not starting from scratch. Use templates based on the latest policies relevant to your models. Prioritize high-risk models for immediate control and monitoring. Automation accelerates governance processes and ROI. Visibility into all models and their compliance is also critical for consistent governance across the organization.
Visibility and Reporting in Model Life Cycles
Jay Combs: How can executives get visibility into the compliance and risk of their model life cycles?
Cristina Morandi: Comprehensive reporting on all model life cycles is essential. Understanding how many models are running, their purposes, compliance scores, and associated risks is critical. We’ve invested heavily in providing this visibility to our customers, helping them answer fundamental questions about their models and governance.
Jay Combs: That’s a great place to stop. We covered a lot about model life cycles today. In the next episode, we’ll discuss reporting and the AI governance score in more detail. Cristina, thank you for joining us. Any final thoughts?
Cristina Morandi: It’s been fun. If I haven’t emphasized enough, no one should be starting from scratch or using manual processes. We can do better.
Jay Combs: Thanks for joining, and thanks for listening, everyone. Subscribe to the podcast for new content regularly. See you next time.